Rock, paper … lizard, Spock?
Year level: 7
Strand: Probability
Lesson length: 50 mins
In this lesson, students compare theoretical and experimental probabilities by completing repeated trials of the game ‘Rock, paper, scissors’ and its extension to include lizard and Spock. Digital simulations and hands-on options for the game are given for variety.
In a prior lesson, students can complete repeated trials of the game ‘Rock, paper, scissors’.
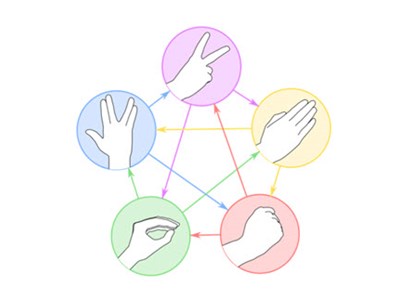
Curriculum information
Achievement standard
By the end of Year 7, students conduct repeated single-step chance experiments and run simulations using digital tools, giving reasons for differences between predicted and observed results.
Content description
Students conduct repeated chance experiments and run simulations with a large number of trials using digital tools; compare predictions about outcomes with observed results, explaining the differences. AC9M7P02
General capabilities
Numeracy
- Understanding chance (Level 5)
Digital literacy
Assessment
The following activity is a suggested opportunity for formative assessment.
Reserve time in the lesson to play the downloadable Matching activity: assessing understanding of the language of experimental and theoretical probability, random and the law of large numbers.
Prerequisite student knowledge and language
It is assumed that students have knowledge of expressing probabilities as a fraction, decimal or percentage.
Language: Equally likely outcomes, chance experiment, relative frequency, trial, experimental probability.
Areas of challenge
- Some students may have misconceptions about what is fair. Although in the whole-class game, from the outset students have an equal chance of being crowned the class champion, students might think the game is unfair if they immediately lose in their first game and so only get one go at the game.
- Students think that a predictable sequence like ‘scissors-scissors-scissors’ or ‘rock-paper-scissors-rock-paper-scissors’ is less likely to win compared to a random sequence (in a game where the opponent chooses randomly), which is a common misconception although each individual random sequence is just as likely or unlikely as these two that show a pattern.
- Some students believe that they can make random choice and think that they are good at making random choices, failing to recognise the inherent bias. Include instruction to guide the student to overcome the misconceptions.
What you need:
Lesson plan (Word)
Teacher's slides (PowerPoint)
Lizard, Spock recording sheet (Word)
Lizard, Spock card cutouts (Word)
Matching activity (Word)
Small opaque bags per group, laptops/tablets for each student
Leaning goals
Learning intention
- We are learning to run a probability simulation.
- We can understand the difference between theoretical and experimental probability and the law of large numbers.
Success criteria
By the end of this lesson, students can:
- calculate theoretical probabilities for equally likely and unlikely outcomes
- use digital tools to simulate probability experiments and calculate experimental probabilities
- explain differences between predicted and observed results and state the law of large numbers and its relevance to the simulation
- explain and justify different game strategies.
Why are we learning about this?
Learning about theoretical and experimental probabilities equips students with an understanding of chance and risk assessment, that helps promote critical thinking and fosters informed decision-making in an increasingly data-driven world. Real-life applications include financial decisions, health choices or daily activities, such as understanding a weather forecast. In the workplace, probability simulations are used in areas including finance, medicine, engineering, climatology and sports analytics.
Learning hook 10 mins
Note: this lesson neatly follows the lesson plan: Rock, paper, scissors; however, both lessons can standalone. Both investigations allow students to explore a familiar game while thinking more deeply about the underlying mathematics and the nature of chance. Use the teacher’s slide to accompany your teaching.
Introduction
Begin by explaining to students that if you play a game against a simple computer simulator, such as, the Rock, paper, scissors game, the computer always chooses its moves randomly.
Refer to slide 2 and ask students to vote for the most effective strategy listed (giving you the best chance of winning) when playing against a randomly choosing computer. For instance:
- Always play the same move: for example, scissors, scissors, scissors ...
- Follow a repeating pattern: for example, rock, paper, scissors, rock, paper, scissors ...
- Make a strategic choice using own judgement.
- Make a random choice.
Follow this with the explanation that if the computer is choosing randomly, it really makes no difference what strategy you follow – you will always have a 1 3 chance of winning.
Now ask students to rank the same strategies listed above if you were to play against an ‘intelligent being’ (or computer) that could analyse your game play (slide 3).
Enable discussion
Against an intelligent opponent, methods 1 and 2 are not a good idea as the opponent would identify the pattern and then beat you every time. The World Rock Paper Scissors Association identifies some strategies to use, including playing ‘paper’ to counter the most common opener ‘rock’, using mirroring of your opponent’s last move or countertactic – playing the move that would beat your opponent’s last move. Another common tactic is win = stay, lose = switch. Hence for advanced players, option 3 is the best strategy, but otherwise option 4 is best: choosing truly random moves.
The research suggests that humans are not good at picking a move at random (for example, people often pick the number 7) as we tend to shy away from apparently repetitious patterns, which actually reduces randomness and therefore increases predictability. Optimal ranking 3-4-2-1 (advanced players) or 4-3-2-1 (novice players).
Learning hook
Refer to slide 4 and ask students to imagine changing the ‘Rock, paper, scissors’ game to include two more elements: lizard and Spock (a very clever humanoid from Star Trek).
Ask students to suggest how lizard and Spock would interact with rock, paper and scissors – who would win each battle and why? What would the new game rules be?
Explore 30 mins
Explain that this is an actual version was invented by Sam Kass and made popular by television series, The Big Bang Theory (slide 5). Demonstrate the game with another student based on the rules:
- Scissors cut paper
- Paper covers rock
- Rock crushes lizard
- Lizard poisons Spock
- Spock smashes (or melts) scissors
- Scissors decapitates lizard
- Lizard eats paper
- Paper disproves Spock
- Spock vapourises rock
- Rock breaks scissors
(Alternatively, use your own student-invented rules, provided Spock and lizard can both beat and be beaten by two other moves, to keep the game fair).
Distribute the Lizard Spock recording sheet and ask students to estimate the probability of winning, losing and tying in this new version of the game (and have them explain their reasoning).
Further explain that we will collect experimental data to support or challenge their theories.
Students play the game in pairs, but this time against a smart computer: Smart rock paper scissors lizard Spock, who sees their previous responses and changes according to a programmed strategy, meaning the computer does not randomly makes choices (slide 6). Therefore, encourage students to try to use a strategy, such as a random or informed choice, to try to beat the computer.
Following this distribute bags and the pre-cut ‘Lizard Spock card cutouts’ so students can choose randomly if they wish.
As before, students will work in pairs, with one student playing against the computer and the other counting the number of trials and recording the results.
The player should stop after 12, 24 and 48 trials, so their partner can record their results on the recording sheet.
The two roles swap, so the second player can play against the computer.
Once each has played and results are collected, students revisit their assumptions on the theoretical probabilities of winning, losing and tying using their experimental evidence.
Summary and reflection 10 mins
Collect and collate the results after 48 trials into a collective tool (a whiteboard or shared spreadsheet), finding the average experimental probability for the class of winning, losing and tying and displaying the data in a divided bar graph, pie chart or bar chart (if using technology).
Explain the law of large numbers: ‘As the number of trials increases, the observed or experimental probability approaches the theoretical or expected probability and ask students how this helps us revise or confirm our estimates on the theoretical probability.’
Lead a discussion on what the actual theoretical probabilities are for this version of this game.
- How would you classify this game? More towards skill or luck? Why?
- What is probability of winning a single game, and what factors might influence this probability?
- Is this game fair?
- How do you approach the game strategically?
- What thought process do you go through when deciding on your moves?
Note: For this version of the game, winning, losing and tying are not equally likely outcomes. There is a 2 5 or 40% chance of each of a win, or loss in a random game and only a 1 5 or 20% chance of a tie. This can be demonstrated by considering the different possibilities. For example, if you play scissors, there are five possible plays by your opponent that means you could tie (opponent plays scissors is one way), win (opponent plays paper or lizard are two ways) or lose (opponent plays rock or Spock are two ways). The same is true for all your other choices as each option gives one way to tie, two ways to win and two ways to lose. Overall, the game is still fair as whenever there is a tie, the game replays and win and loss are equally likely. This suggests that if students are winning significantly less than 40% of games, then they are using ineffective strategies as the computer has made better choices and won more often than expected.